BACK TO ARTICLES
Are You Struggling to Build a Reliable Commercial Data Infrastructure to Support Your First Launch?
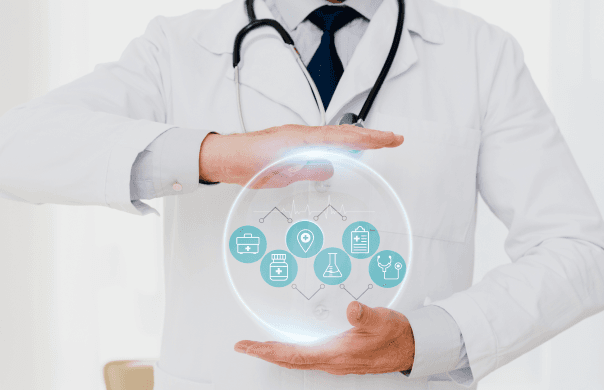
For pharmaceutical companies, the first drug launch is critical. Without a strategic and technical blueprint, this launch can quickly become a major challenge. What can pharmaceutical manufacturers do to ensure a high degree of success without risking millions of dollars?
Commercial teams in pharma companies actively seek data-driven answers to help build a reliable launch plan. To support this, technology teams must determine the right technology stack, which modules to outsource, and the steps to prioritize. They often struggle to know where to start and how to ensure success on the first try. These high-impact decisions need to account for scalability, data security, and governance, adding to the complexity of the launch strategy.
Recently, a pre-commercial oncology brand, struggling to build a reliable cloud-based commercial data infrastructure, reached out to ProcDNA for help. ProcDNA experts assisted the company in avoiding common mistakes, saving millions of dollars in investment, and accelerating their decision-making for a clear and reliable commercial data infrastructure strategy.
1. Create a Detailed Roadmap
Our first step was to involve the client’s business teams in creating a roadmap for short-, mid-, and long-term implementation. This included defining the business questions that needed answers and establishing success metrics. Often, teams start by creating a standalone analytics sandbox to analyze sales and claims data, thinking this will answer their business questions. However, this approach can introduce unnecessary silos into the process. Analytics teams tend to get accustomed to the sandbox environment, making it difficult to adapt when the final infrastructure is ready. To avoid this pitfall, we used our proprietary EDL framework and set up the sandbox within the Azure Data Lake environment.
The client also wanted to create a digital-native marketing strategy and keep all marketing data in-house. With these directives, the company was able to prepare for the development of a robust data infrastructure. We helped design the Azure data lake to house all types of marketing content and integrated DataBricks into the architecture to enable advanced data science capabilities. This ensured real-time communication with the MarTech platforms.
2. Adopt Modular, Best-of-Breed Platforms
The analytics and advanced data science market offers a wide range of tools to suit various needs. We helped our life sciences clients filter out the noise and choose the best tools for their requirements. Our goal was to source best-of-breed tools that integrate seamlessly into the overall data infrastructure. A highly modular data architecture allowed the client to combine licensed tools with open-source components, which could be plugged in and out as needed. To avoid complications in the application layer, most integrations with analytics and visualization tools were done using APIs.
3. Reduce Waste with Common Data Layouts (CDLs)
To generate consistent results across different functions and reduce operational overheads, ProcDNA experts introduced Common Data Layouts (CDLs). These CDLs allowed teams to create reusable assets and fostered a culture of reusability within the organization. We also helped the client define a list of Critical Business Questions (CBQs). The CDLs were exposed to the application layer using a Role-Based Access Control (RBAC) system, which controlled the level of data access for analytics sandboxes, advanced analytics, and visualization layers.
4. Design and Build to Scale
During the lifecycle of a commercial product launch, large volumes of data pour in from various channels over a short period of time – product sales, patient claims, call activity, sampling, speaker bureaus, branded/unbranded websites, emails, digital campaigns, and more. It is recommended to design pipelines in advance with dummy data, but companies must also consider scalability to handle these parallel data streams, which can flood systems with data in large spurts. Data infrastructure teams must be familiar with the varied data sources specific to the pharmaceutical industry, treating it as more than just another IT project.
Testing pipelines with realistic data attributes and volumes is essential. Our experience with life sciences companies shows that the lack of real-data testing often leads to failure in meeting critical launch KPIs weeks after the product launch.
Conclusion
By following these four best practices for building a robust on-cloud commercial data infrastructure, life sciences companies can better support new brand launches. A sophisticated data infrastructure, built to scale, empowers data and commercial teams to generate valuable insights, freeing them from worrying about data operations.
Read More Articles